
Measuring Deforestation From Space
Testing a new Google Earth Engine tool that uses satellite imagery to detect changes in land use/land cover in Maine's northern forests
Land use/land cover (LULC) describes how raw satellite imagery is classified into land use, or how human activities change the landscape (i.e. agriculture, urban development), and land cover, the natural features of the landscape (i.e. forest, wetland, desert). Observing LULC change is an useful way of analyzing changes in habitat for critical species, which is why the Defenders of Wildlife has developed a new tool to measure LULC change.
Remote sensing involves using satellites to make observations about the Earth. Often, visible light is collected to make aerial images of the Earth, but satellites collect other types of light, such as infrared, to make more in-depth analysis of Earth. These different wavelengths of light can be combined mathematically to create a spectral index, which can provide more information about the landscape. A typical example of a spectral index is NDVI (normalized difference vegetation index), which combines red and infrared light to tell how vegetated an area is. The Defender’s tool measures change in 5 spectral indices to tell the likelihood of any given pixel representing real change. For more about remote sensing and NDVI, click here.
Google Earth Engine (GEE) is a relatively new, free-to-use geographic information system (GIS) developed by Google that focuses on using remotely sensed data, such as satellite imagery, and Google’s cloud computing abilities to simultaneously offer high resolution data and large scale analysis (Gorelick et al., 2017). The imagery used by the tool is from the Sentinel-2 satellite, launched by the European Space Agency in 2015, which combines an unprecedented global scale with high resolution 10x10m pixels (ESA, 2016). GEE allows easy access and analysis of Sentinel-2 data, allowing small-scale LULC changes to be analyzed on a wide scale.
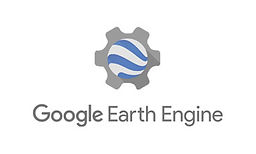
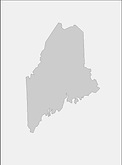
Location Map
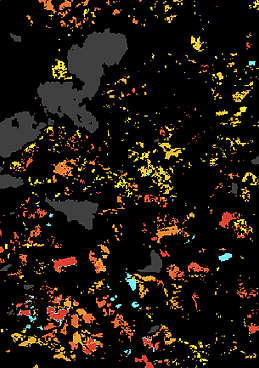
This study focused on testing this tool for a specific habitat disturbance: logging. Each year, 46-58 million square miles of forest are lost due to a variety of natural and anthropogenic factors (WWF, 2017). Logging, especially clear-cut logging, can have a large impact on these forest environments. The northwoods region of Maine, despite being known for its “wild” nature, is heavily used as a commercial forest, and is crisscrossed by a network of logging roads connecting large patches of clear-cut forest (Breen, 1988). This study area, located near Greenville, ME and Moosehead Lake, has had several large areas of forest cut in the last year, while remaining relatively undisturbed by other types of disturbances, like residential development or wildfires, making it a good testing ground for the Defender’s of Wildlife LULC change detection tool.
The pattern of deforestation in the study area over the last 20 years (Hansen et al., 2013; Retrieved from https://earthenginepartners.appspot.com/science-2013-global-forest)

Observing Change
Try and find all of the logged areas by sliding between the before and after images!
All areas of change were identified by manual comparing the two images. Green polygons are areas of "real" change (logging), while red are unchanged areas. The shapes are identical to ensure 1:1 sampling.
Try and find all of the logged areas by sliding between the before and after images!
Results
Compare the change image made by the IW tool and the polygons manually thought to be real/not real change!
Areas of red/yellow show higher likelihoods of change, while blue/green show lower likelihoods of change.
The IW change detection image is symbolized using NBR, NDVI, and RCVmax as R,G,B bands.
Change Detection Image
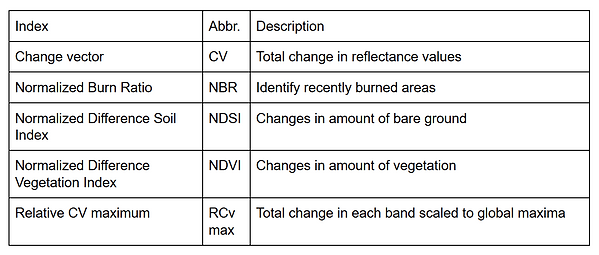
The study area was manually classified, with all areas of change due to logging being bounded by a “real” polygon. To ensure 1:1 sampling, identical “not real” polygons were drawn over areas near the real polygons with no visible change. These polygons samples pixels, which were summarized in spreadsheets with their associated tool outputs. The IW tool outputs Z-scores for every pixel indicating the likelihood that pixel represents change for each spectral index.


Boxplots of the Z-scores for each index for pixels marked as real/not real in the manual classification
The tool requires the user to set manual thresholds to determine what outputted Z-scores represent real and not real change. Z-score thresholds were set to maximize the difference between the true postive and false negative percents, not to maximize the true positive accuracy (and minimize the false positive error), because often the lowest values for false positive error would simultaneously result in very high false negative values. Thresholds were generated using boxplots of the Z-score distributions, the mean and standard deviation of the distributions, and trial and error.

The accuracy of the tool for each spectral index was calculated based on comparing the results of the manual classification (actual) and the tool’s Z-score outputs (measured). The true positive and true negative are highlighted in green. The upper right corner is the false positive error (not real change pixels identified as real), and the lower left corner is the false negative error (real change pixels identified as not real).
The most accurate index by far was the RCVmax, with false postive of 2% and false negative of 12%. NBR was the next best, with 25% false positive and 0% false negative. CV, NDSI, and NDVI are all around the same level, with barely better than 50% true positive rates, although NDVI only has 18% false negative error. The three best-performing indices, RCVmax, NBR, and NDVI were used to symbolize the IW change image above. When only the best-performing indices are used, the IW can be a good indicator of change, but performance is very uneven across the 5 indices. RCVmax alone performs so well, that the tool could be better off in certain situations by only considering this metric.
There a several sources of error which could also be affecting the results. Images were not taken during the same season, although general leaf cover was similar and cloud cover was minimal. The nature of the disturbance is also somewhat heterogeneous; blocks of forested land are not completely clear cut and many areas still contain low-lying vegetation that the tool is marking as tree cover, which could explain the relatively high NDVI false postitive error when compared to its false negative error. Likewise, the burn ratio did a good job of identifying the tree cover to bare ground transition, although none of these areas have appeared to actually burn, which could be problematic in forest habitats that are more susceptible to wildfires when differentiating between disturbance types. The Z-score thresholds were set through a mixture of general observation and trial and error, and would be better served if estimated by an optimization algorithm. Lastly, the tool results are compared to a manual classification, which is assumed to be a perfectly accurate benchmark. While effort was made to scan for logged areas on a variety of scales, potential logged areas could have been missed or accidentally included in not real polygons, and polygons could also not have perfectly bounded areas of change.
Management Implications
86% of Maine’s total area is considered “commercial forest”, with almost half of logging being conducted with “clear cut” practices (Breen, 1988). Clear cutting continues today, with large logging companies skirting regulations designed to minimize the practice (Bayly, 2014). Clear cutting has been shown to have generally adverse effects on the environment, from nutrient loss (Bormann et al., 1967) to increased runoff and stream discharge (Jones and Grant, 1996). Most importantly for the Defenders of Wildlife, clear cutting can lead to fragmentation of forest and habitat loss, with large effects on generalist and interior species (Bender et al., 1998). Species of interest to the Defenders of Wildlife in Maine that stand to be impacted by habitat fragmentation include the Canada lynx, fisher, and black bear, as well as efforts to reintroduce grey wolf populations (www.defenders.org). Logging companies have cited lowered restrictions on clear cutting as aiding profits (Bayly, 2014), a tempting arrangement for the state, as these companies are one of the few employers in these fairly remote regions. Therefore buying the millions of privately owned acres back as conservation lands or increasing regulations on clear cutting are probably unfeasible, especially given Maine’s current political climate. A more pragmatic approach could be increased monitoring and enforcement of existing regulations and limiting the expansion of clear cut areas, possibly supported by remote sensing methods to help access the most remote corners of Maine’s northwoods region.
Works Cited
Bayly, J. (2014, February 2). Freed from state clear-cut restrictions, Maine’s largest landowner says it’s doing better forestry. Bangor Daily News.
Bender, D. J., Contreras, T. A., & Fahrig, L. (1998). Habitat Loss and Population Decline: A Meta-Analysis of the Patch Size Effect. Ecology, 79(2), 517–533.
Bormann, F. H., Likens, G. E., Fisher, D. W., & Pierce, R. S. (1968). Nutrient Loss Accelerated by Clear-Cutting of a Forest Ecosystem. Science, 159(3817), 882–884.
Breen, B. (1988, November 8). Maine woods - wilderness or working woodlot? Network of logging roads gives the public access to once remote timberlands. Christian Science Monitor.
Defenders of Wildlife | Protecting Native Animals and Their Habitats. (n.d.). Retrieved October 25, 2017, from http://www.defenders.org/
ESA. (2016). Introducing Sentinel-2. Retrieved December 4, 2017, from http://www.esa.int/
Gorelick, N., Hancher, M., Dixon, M., Ilyushchenko, S., Thau, D., & Moore, R. (2017). Google Earth Engine: Planetary-scale geospatial analysis for everyone. Remote Sensing of Environment.
Hansen, M. C., Potapov, P. V., Moore, R., Hancher, M., Turubanova, S. A., Tyukavina, A., … Townshend, J. R. G. (2013). High-Resolution Global Maps of 21st-Century Forest Cover Change. Science, 342(6160), 850–853.
Jones, J. A., & Grant, G. E. (1996). Peak Flow Responses to Clear-Cutting and Roads in Small and Large Basins, Western Cascades, Oregon. Water Resources Research, 32(4), 959–974.
WWF. (2017). Forest Habitat. Retrieved December 4, 2017, from https://www.worldwildlife.org/habitats/forest-habitat
Images
http://d.ibtimes.co.uk/en/full/1509489/earth-day-2016.jpg
https://earthengine.google.com/static/images/GoogleEarthEngine_Grey_108.png
Sentinel-2 satellite imagery courtesy of Google Earth Engine
Data Download
Google Earth Engine
Timelapse: https://earthengine.google.com/timelapse/
Explorer: https://explorer.earthengine.google.com/#workspace
Code Editor: https://code.earthengine.google.com/